ARTICLE
Holistic Knowledge-driven corporate Management – GenAI and Digital Twins
A productive industrialized economy like Germany needs besides a well-educated work force a functioning supply chain, cheap energy, and a high level of technological productivity to be competitive in the international markets. That is, innovation plays a major role.
It is a common place that the development and application of AI methods will be a key enabler driving innovation across the board in the future. In industrial settings, however, it will be crucial to provide AI readiness before applying AI methods. The latter relates to the fact that corporate heterogeneous data stored and maintained in various repositories within and across business processes are not ready to be used. GenAI technologies applied on corporate data to gain insights in many cases fails due to AI hallucination. GenAI always delivers an answer, but the result might not be appropriate. To gain useful results corporate data needs to be transferred into business knowledge. This is a prerequisite for the development of alternative courses of action which intrinsically relates to competence and smart decisions leading to the much-needed competitive edge of a company. This idea is very well captured by the concept of the “knowledge stairs” developed by Klaus North and extended by Udo Jauernig and Conweaver. Holistic knowledge-driven corporate management is the interface between business pull (strategic knowledge management) and technology push (operational knowledge management).
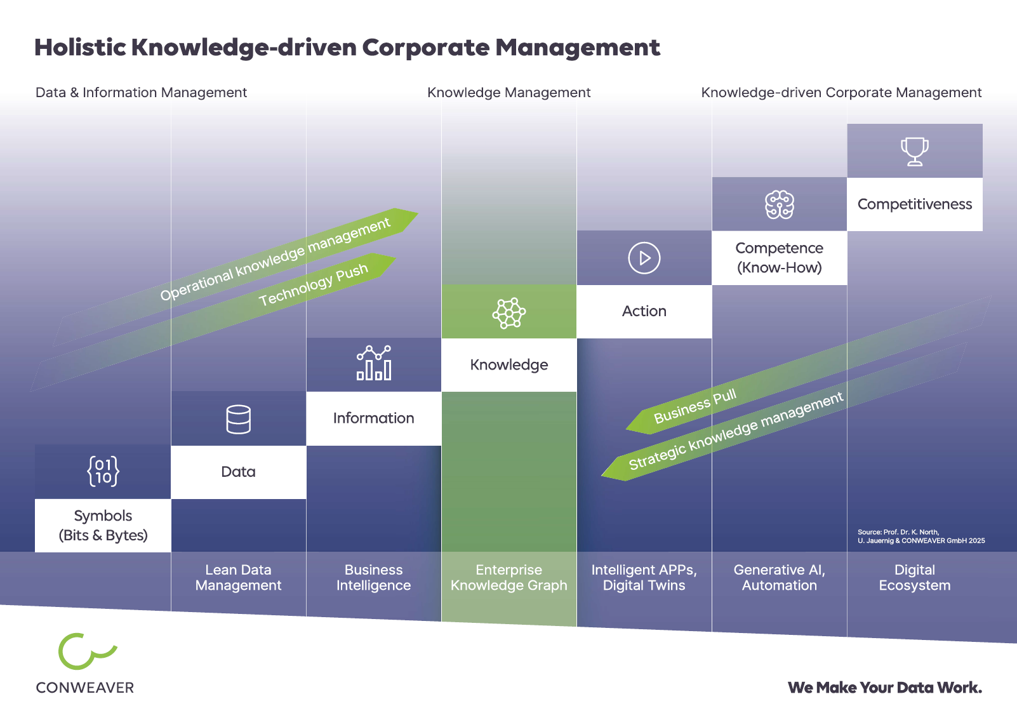
Data Democratization & Speed of Innovation
In recent years one aspect has become particularly interesting to observe. It is related to the fact that IT organizations are not fast enough to serve the needs of their internal business customers. Long processing queues require customers to queue, many at the end, resulting in long lead times for their turn. This is one of the reasons why data democratization is important, another one relates to the fact that business knowledge reflects the tailored character of the business processes themselves. Special expertise is bound to knowledge domains required to execute the processes properly. And such knowledge domains are spread across the company: for example, engineering, production engineering, production, supply chain, sales, purchasing, etc. and even subdomains of such fields of expertise. Because software plays a major role engineers become software experts. These circumstances require customers to follow federated knowledge management architectures where domain knowledge is captured using domain-specific ontologies – an important means for standardization and thus automation of processes. Based on reusable federated knowledge structures and self-services, IT-savvy engineers are enabled to design knowledge-based applications without need for repetitive data modeling and transformations and thus meet customer demand quickly.
Graph Mesh
A federated knowledge architecture can best be represented using domain knowledge graphs computed from the data in the authoring systems in regard to domain ontology modeling. In other words, there is not just one single enterprise knowledge graph covering the knowledge of an enterprise but a set of graphs evolving out of local demands. To ensure the combined use of domain graphs at the enterprise level the local graphs need to be linked together to provide a continuous digital thread. The resulting structure is what we call a graph mesh. Based on the Conweaver Linksphere Platform this is illustrated in the architecture depicted below.

The graph mesh provides standard APIs so that access to the graph is easily possible for business intelligence, advanced analytics, extended reality digital twins and GenAI. All these applications may benefit from the business contexts delivered by the graph mesh.
Graph Mesh, GenAI, and Digital Twins
As outlined in the beginning enterprise knowledge graphs are GenAI enablers because they prevent it from hallucination. Why? Because GenAI does not need to learn the “raw” data in the authoring systems, but it needs to able to query the graph mesh representing the corporate knowledge. This makes it easy for business users to query the enterprise knowledge using natural language interfaces. It even overcomes the limitations of GUIs which are bound to serving use case-specific information access strategies. Using GenAI to query the graph mesh it is possible to demand the following get sensible answers for the query below. It would be quite cumbersome to get the results using Google-like graph-based search engine.

Digital Twins such as “as designed”, “as produced”, “as maintained” etc. occur along the complete product lifecycle. An important requirement for digital twins to be had is that the business context by means of connected data is required as input. And this context is provided by the graph mesh too. In other words, once you have a graph mesh architecture in place you can derive the context for any kind of digital twin from it (depending on the coverage of the graph mesh) – build it once use it many times.