ARTICLE
GenAI in Business
With AI in general and GenAI in particular on everyone's lips, enterprises are trying to figure out how to use GenAI to improve their business. As with many hyped technologies, there is a lot of uncertainty in the market on both the customer and vendor side about how the technology can be applied to deliver business value.
Looking back to the early 2000s, when Google introduced its Google Search Appliances into the enterprise environment, expectations were high because Google had revolutionized Internet search. It seemed that this silver bullet could be used to deliver similar benefits in the enterprise. However, this turned out to be an illusion. Full-text search engines in the enterprise are, with all due respect, only useful for finding out what's on the menu in the cafeteria. Of course, it is important to search for patents, documentation, etc. We know we’re exaggerating, sorry, apologies 😊.
Business content is related to the business capabilities and their processes
However, the real reason why full-text search engines are of limited use in an enterprise context is that they only index text documents.
What they don't consider is that the real knowledge of the enterprise is based on the business objects created and maintained in the various functional business capabilities and their processes. Every company has business capabilities such as sales, engineering, manufacturing, and so on. Each company creates and maintains its own unique core business objects. The "customer" is the core object of the sales processes, and similarly the "product" or "system" is the core object of the product life cycle, while in manufacturing the "production machines" and the "products produced" are the core objects.
These core objects change over time, as in the case of manufacturing, by collecting additional data related to business activities such as requirements engineering, modeling, simulation, sensor data analysis, and so on. As a result, they add value at different stages of the system lifecycle, complement each other, and change state over time. To keep track of these changes, it is important to derive digital twins at any time. Digital twins can be thought of as abstractions of technical artifacts created at any point in the system lifecycle.

The Structure of Business Data on a High Level of Digitalization
The creation and maintenance of core business objects typically takes place as part of authoring processes in dedicated authoring systems such as ERP, CRM, PDM, MES, SCM, CAE, PLM and many other categories of systems. Such systems typically contain structured data such as database records or object-oriented structures.
In addition, there are data lakes or lake houses as storage containers for large amounts of unstructured data such as field data. The fact that different industries have developed structured data systems reflects an already high level of functional digitization. We would argue that industries that lack such structured, data-driven processes are underdeveloped.
For example, a decade or more ago, the construction industry relied heavily on documents stored in file systems. Today, Building Information Management (BIM) and related authoring systems are an established standard. In these "structured environments", the main obstacle is related to the silo nature of the functional authoring systems. The overarching business processes lack business context because the data is not connected between the functional authoring systems and their processes.
This is where graph technology comes in, providing a native way to represent dependencies between business objects. By automatically linking data across functional authoring systems, business context can be provided in a very elegant and cost-effective way throughout the product lifecycle. And when combined with high-end search technology, it enables on-demand retrieval and coherent representation of data distributed across multiple data systems and processes.
For example, engineers can now be informed of projected costs over the next few years as they develop the product. For example, purchasing data can be accessed online to create accurate product quotes in the early stages of development. It is also possible to determine during development which facility can produce the artifact and who might be the cheapest supplier in the region. Similarly, connected product data can be a prerequisite for accurate sales forecasting.
These examples require the data connection of different business capabilities and processes. In the first and last examples, engineering data must be connected to purchasing data; in the second example, engineering data must be connected to both manufacturing and supply chain data. In most companies we have encountered, such cross-process data access remains a distant dream because companies still lack cross-process business context as the highest level of data management via "business knowledge representation". These examples also show that CONWEAVER, as a software vendor, understands the need to deeply comprehend the interactions of our customers' business processes in order to deliver sustainable value.

Business Knowledge Graphs and the Industrial Metaverse
Our experience and collaboration with our industrial customers suggest that processes are likely to be connected by locally distributed, large-scale Business Knowledge Graphs.
However, a critical requirement is that these local graphs must be connected so that a so-called digital thread can connect them as if they were a single graph. Federated graphs, as we call them, address business owners’ desire for data ownership and data democratization. But it is also, in my opinion, a mandatory prerequisite for the establishment of the "industrial metaverse", as the Siemens CEO Roland Busch propagated at the last conference in Las Vegas. However, it requires more technology than just the connecting data part that we can provide with our Business Knowledge Graph technology.

There is the need to derive digital twins and their evaluation based on field data and others such as production data using machine learning techniques, there is immersivity, three-dimensional haptic user experience, and there is GenAI, designed to support the expertise, creativity and knowledge of engineers during the design process, but also to dramatically expand the number of consumers of valuable business content to support the entire product lifecycle.

GenAI and Business Knowledge
Like Google Search, GenAI started with large, publicly available text bases. At the heart of GenAI are Large Language Models (LLMs), which can be used as the core technology of intelligent chatbots such as ChatGPT (provided by OpenAI). They have demonstrated how LLMs can be used to automatically create summaries, suggest program code, generate music and video, provide language translation, and support other applications.
In addition to Open AI, there are several other providers of LLMs, including Facebook with LLaMA, the German provider Aleph Alpha with Luminous, and the French provider Mistral with 7B LLM. LLMs are typically trained to understand natural language, which makes them very valuable. But... Here comes the “but”... As we tried to argue above, business content is typically not represented by unstructured text data, but rather is stored in distributed data systems across processes and sometimes even across organizational boundaries. These data systems have their own query languages, and then there is the silo problem that leads to data disruption.
So far, GenAI is limited to working with text data if you want to support the business processes based on the business capabilities. Therefore, it makes a lot of sense to bring together two things that complement each other well - business knowledge (represented by composite graphs) and algorithmic AI (represented by GenAI and LLMs). The main advantage is that GenAI can operate on large, reliable, interconnected knowledge bases that represent the core tasks of the company, thus avoiding the problem of AI hallucination.
We are in the process of implementing projects that combine the two technology approaches to deliver real business value to customers. Below is an example of how such an approach might work. You could formulate a fairly complex query such as, “Do we have test cases that are not canceled related to requirements that are canceled in the ThunderStrike project?” Using a Google-like search interface to query a Linksphere (CONWEAVER´s Low Code Large Graph Platform) based engineering knowledge graph it would require a complex browsing process to get to the result set (if you get there at all) like the one shown below. By combining ChatGPT with the Linksphere graph, the user can use his own natural language to formulate the query, and the LLM in turn knows how to query the reliable knowledge base and return the result without hallucination. In general, a major advantage is that GenAI, unlike graphical user interfaces for retrieval, allows a very flexible way to access the needed data.
.png)
From the above discussion, we conclude that for engineers who need to design products, the transition from today's search-based graph-based information access to AI-based intelligent suggestion will take place in three steps. The above example shows the second step, which already works quite well. Again, there are a number of stumbling blocks that need to be addressed in our development, but we consider this to be a short-term goal.
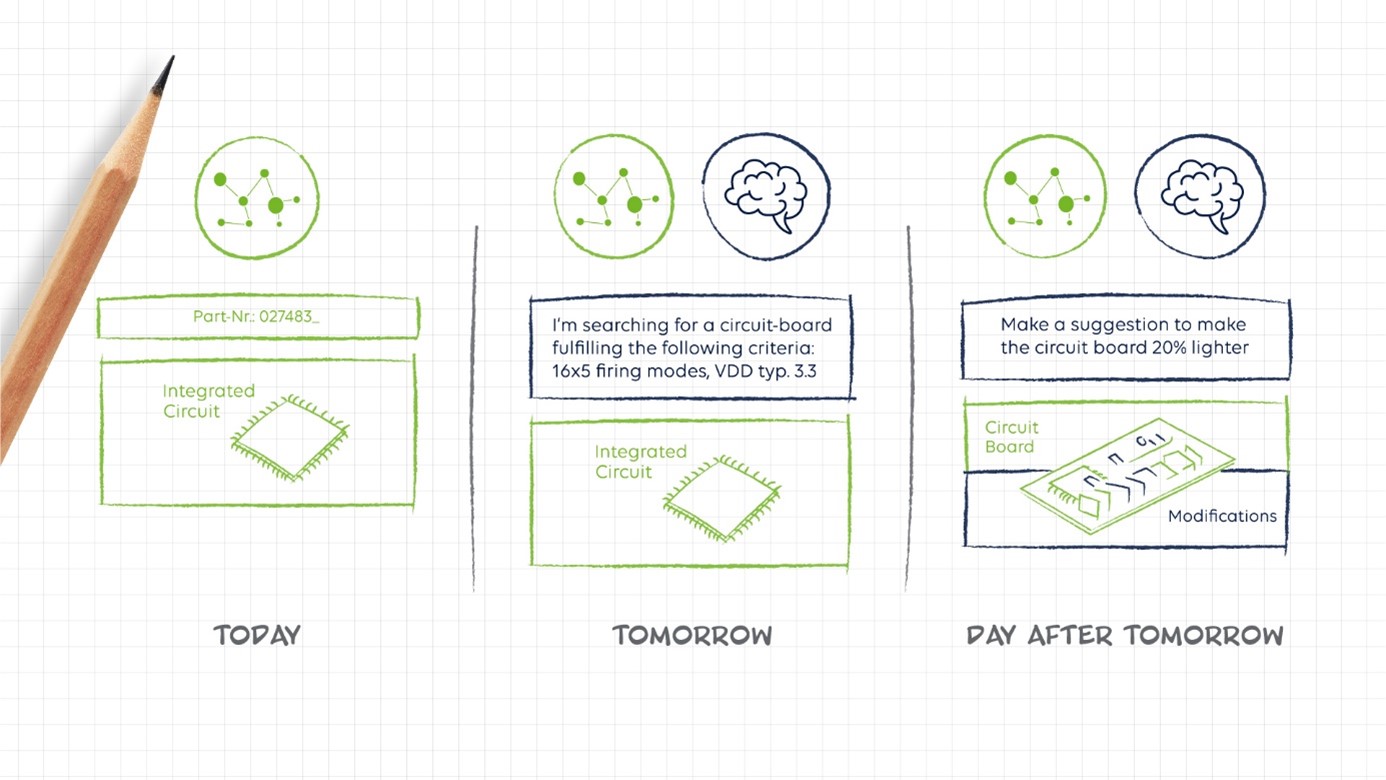
The Outlook
Business Knowledge Graphs together with GenAI will become the brain part of the company’s digital central nervous system connecting and processing data and information from different functional Knowledge Graphs.
As CONWEAVER, we support our customers in organically developing this graph-based digital central nervous system to enable them to make the best use of algorithmic AI technologies.
One aspect we would like to address in a follow-up article is related to the capability of Knowledge Graph technology to support data-driven holistic business management. We will show how such a technical approach can help closing the control loops at all levels within and across business functions, and moreover, how linking data and information with the companies’ knowledge and capabilities can facilitate collaboration and increase competitiveness in challenging and dynamic markets.